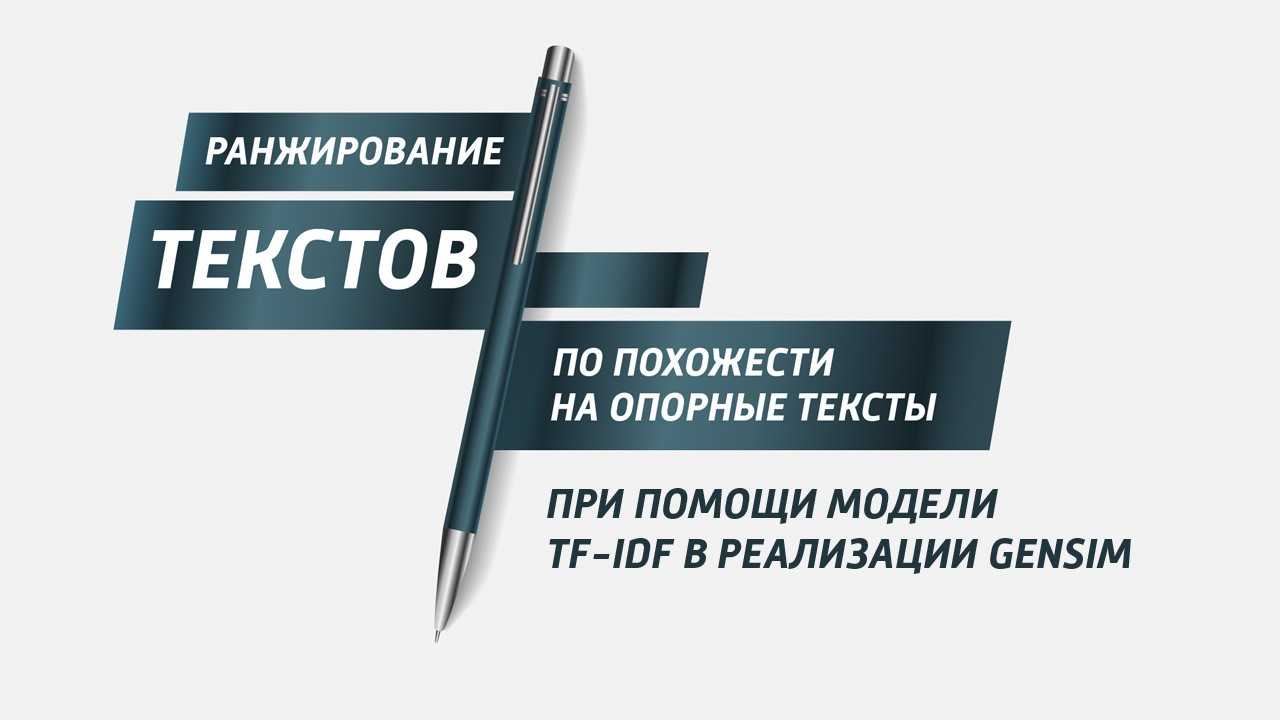
テキストの検索条件が複雑すぎて正規表現ではうまくいかないことがあります。そのような場合、MLが助けになります。テキストのリストから私たちに最も適したものを選択すると、他のすべてのテキストとこのテキストとの類似性を見つけることができます。類似性は数値的な尺度であり、高いほどテキストが類似しているため、このパラメーターで降順で並べ替えると、選択範囲から最も適切なテキストが表示されます。
. http://study.mokoron.com/ csv . , , . , , pandas :
import pandas as pd
import re
from gensim import corpora,models,similarities
from gensim.utils import tokenize
df = pd.read_csv('positive.csv',sep=";",names = [1,2,3,"text",4,5,6,7,8,9,10,11])[["text"]]
list(df.head(5)["text"].values)
['@first_timee , , :D )',
', - . :D',
'RT @KatiaCheh: ) !!!',
'RT @digger2912: " , 2 , " :DD http://t.co/GqG6iuE2…',
– . . , :
regex_queries = [".*",".*",".*",'[^--][^--]']
for word in regex_queries:
df[word] = df["text"].str.count(word,flags=re.IGNORECASE)
.* . [^--] « , ». regex. str.count pandas , . re.IGNORECASE regex, , .
:
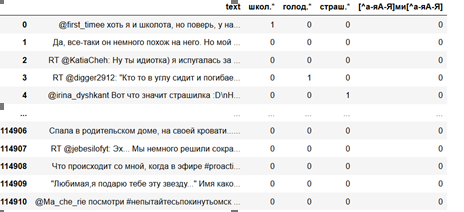
, , . , « », « », «.*» «.*» , .
doc2bow genism, , , , .
, , « ». , , . 5 , , .
texts_to_compare = list(df.head(5)["text"])
['@first_timee , , :D )',
', - . :D',
'RT @KatiaCheh: ) !!!',
'RT @digger2912: " , 2 , " :DD http://t.co/GqG6iuE2…',
'@irina_dyshkant :D\n , , , - :D']
. , . :
, «», «», «» . . NLTK
. python . , , gensim.
, . , . pymystem3 , .
, . , «» «». . pymorphy .
def tokenize_in_df(strin):
try:
return list(tokenize(strin,lowercase=True, deacc=True,))
except:
return ""
df["tokens"] = df["text"].apply(tokenize_in_df)
df.head(5)["tokens"].values
array([list(['first_timee', '', '', '', '', '', '', '', '', '', '', '', 'd', '', '', '', '']),
list(['', '', '', '', '', '', '', '', '', '', '', '', '', '', 'd']),
list(['rt', 'katiacheh', '', '', '', '', '', '', '']),
list(['rt', 'digger', '', '', '', '', '', '', '', '', '', '', '', '', '', '', '', '', '', '', '', '', '', 'dd', 'http', 't', 'co', 'gqg', 'iue']),
list(['irina_dyshkant', '', '', '', '', 'd', '', '', '', '', '', '', '', '', '', '', '', '', '', '', 'd'])],
dtype=object)
gensim.tokenize: lowercase=True, deacc=True.
, :
dictionary = corpora.Dictionary(df["tokens"])
feature_cnt = len(dictionary.token2id)
dictionary.token2id
{'d': 0,
'first_timee': 1,
'': 2,
'': 3,
'': 4,
'': 5,
'': 6,
'': 7,
'': 8,
'': 9,
'': 10,
'': 11,
'': 12,
'': 13,
'': 14,
'': 15,
'': 16,
'': 17,
'': 18,
'': 19,
…
. , . , ( bow – bag of words – ). — « : ».
corpus = [dictionary.doc2bow(text) for text in df["tokens"]]
corpus
[[(0, 1),
(1, 1),
(2, 1),
(3, 3),
(4, 1),
(5, 1),
(6, 1),
(7, 1),
(8, 1),
(9, 2),
(10, 1),
(11, 1),
(12, 1),
(13, 1),
(14, 2),
(15, 1),
(16, 1),
…
, , , , , .
tf-idf. TF-IDF TF — term frequency, IDF — inverse document frequency, . , , .
tfidf = models.TfidfModel(corpus) index = similarities.SparseMatrixSimilarity(tfidf[corpus],num_features = feature_cnt)
, .
for text in texts_to_compare:
kw_vector = dictionary.doc2bow(tokenize(text))
df[text] = index[tfidf[kw_vector]]
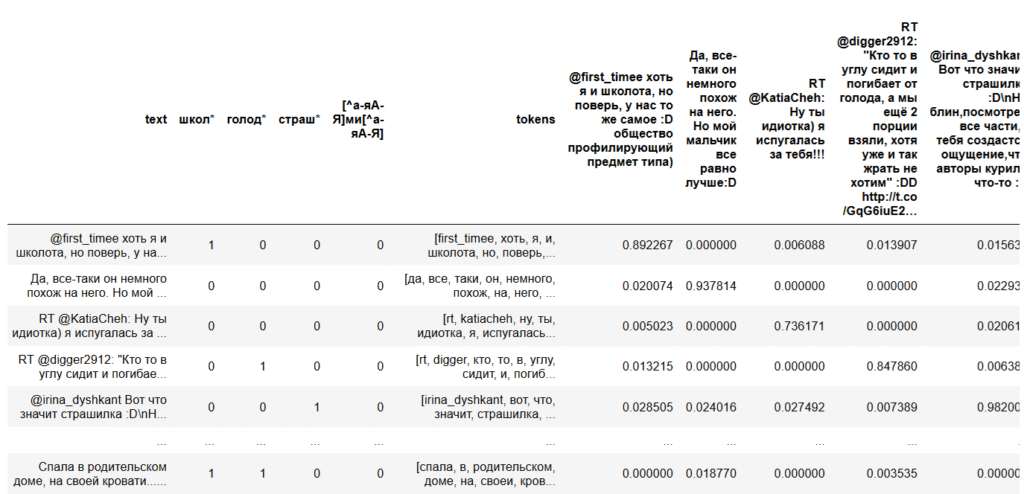
, . , , , .
df["sum"] = 0
for text in texts_to_compare:
df["sum"] = df["sum"]+df[text]
for word in regex_queries:
df["sum"] = df["sum"]+df[word]/5
, .
df["sum"].value_counts(bins=5)
(-0.0022700000000000003, 0.254] 113040
(0.254, 0.508] 1829
(0.508, 0.762] 31
(0.762, 1.016] 7
(1.016, 1.269] 4
python , excel:
df[df["sum"]>0.250].to_excel(" .xlsx")
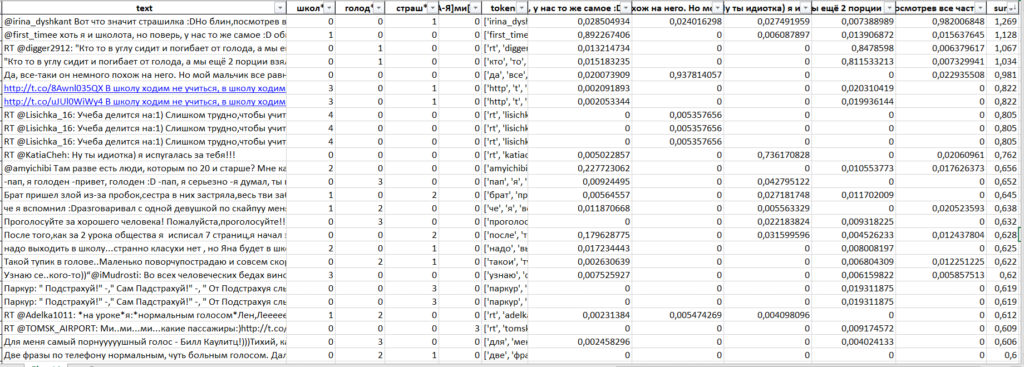
Excel, .
, (, , ):
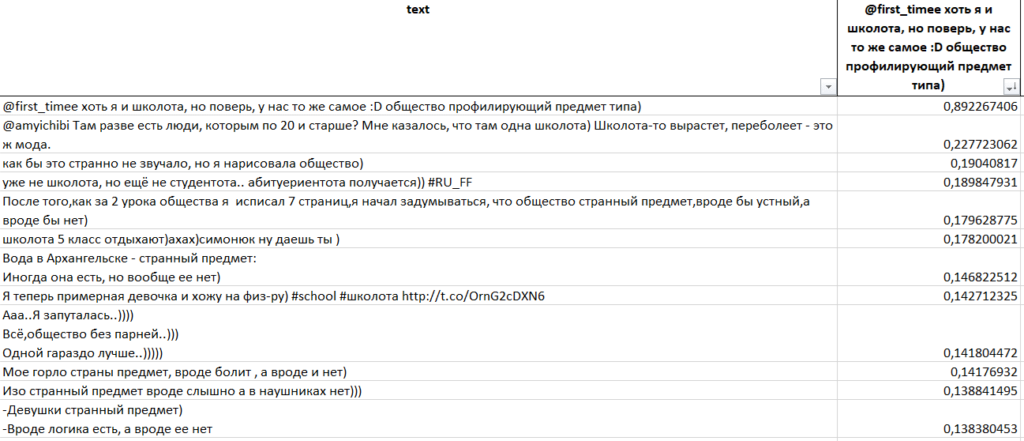
, «», «» «», , .
, texts_to_compare, .