コンピュータビジョンは、複雑な人間の視覚システムの一部を再現することに焦点を当てたコンピュータサイエンスの分野であり、コンピュータが画像やビデオ内のオブジェクトや人を識別して処理できるようにします。コンピュータビジョンの初期の実験は1950年代に始まり、1970年代に印刷されたテキストと手書きのテキストを区別するために最初に商業的に使用されました。今日、コンピュータビジョンアプリケーションは飛躍的に成長しています。この記事では、コンピュータビジョンを使用して道路標識を認識する方法の例を示します。

, Kaggle: GTSRB — , (IJCNN) 2011. 50 000 43 . : , - .
.
import os
import matplotlib
import numpy as np
from PIL import Image
from tensorflow.keras.preprocessing.image import img_to_array
from sklearn.model_selection import train_test_split
from keras.utils import to_categorical
from keras.models import Sequential, load_model
from keras.layers import Conv2D, MaxPool2D, Dense, Flatten, Dropout
from tensorflow.keras import backend as K
import matplotlib.pyplot as plt
from sklearn.metrics import accuracy_score
«train», 43 . : data labels. , , .
data = [] labels = []
, os data labels. PIL.
for num in range(0, classes):
path = os.path.join('train',str(num))
imagePaths = os.listdir(path)
for img in imagePaths:
image = Image.open(path + '/'+ img)
image = image.resize((30,30))
image = img_to_array(image)
data.append(image)
labels.append(num)
30×30 data labels.
numpy .
data = np.array(data) labels = np.array(labels)
- (39209, 30, 30, 3), , 39 209 30×30 , 3 , ( RGB).
print(data.shape, labels.shape)
(39209, 30, 30, 3) (39209,)
sklearn
train_test_split()
, 80% 20% . .
X_train, X_test, y_train, y_test = train_test_split(data, labels, test_size=0.2, random_state=42)
print(X_train.shape, X_test.shape, y_train.shape, y_test.shape)
(31367, 30, 30, 3) (7842, 30, 30, 3) (31367,) (7842,)
, .
def cnt_img_in_classes(labels):
count = {}
for i in labels:
if i in count:
count[i] += 1
else:
count[i] = 1
return count
samples_distribution = cnt_img_in_classes (y_train)
def diagram(count_classes):
plt.bar(range(len(dct)), sorted(list(count_classes.values())), align='center')
plt.xticks(range(len(dct)), sorted(list(count_classes.keys())), rotation=90, fontsize=7)
plt.show()
diagram(samples_distribution)

, , , .
def aug_images(images, p):
from imgaug import augmenters as iaa
augs = iaa.SomeOf((2, 4),
[
iaa.Crop(px=(0, 4)),
iaa.Affine(scale={"x": (0.8, 1.2), "y": (0.8, 1.2)}),
iaa.Affine(translate_percent={"x": (-0.2, 0.2), "y": (-0.2, 0.2)}),
iaa.Affine(rotate=(-45, 45))
iaa.Affine(shear=(-10, 10))
])
seq = iaa.Sequential([iaa.Sometimes(p, augs)])
res = seq.augment_images(images)
return res
def augmentation(images, labels):
min_imgs = 500
classes = cnt_img_in_classes(labels)
for i in range(len(classes)):
if (classes[i] < min_imgs):
add_num = min_imgs - classes[i]
imgs_for_augm = []
lbls_for_augm = []
for j in range(add_num):
im_index = random.choice(np.where(labels == i)[0])
imgs_for_augm.append(images[im_index])
lbls_for_augm.append(labels[im_index])
augmented_class = augment_imgs(imgs_for_augm, 1)
augmented_class_np = np.array(augmented_class)
augmented_lbls_np = np.array(lbls_for_augm)
imgs = np.concatenate((images, augmented_class_np), axis=0)
lbls = np.concatenate((labels, augmented_lbls_np), axis=0)
return (images, labels)
X_train, y_train = augmentation(X_train, y_train)
.
print(X_train.shape, X_test.shape, y_train.shape, y_test.shape)
(36256, 30, 30, 3) (7842, 30, 30, 3) (36256,) (7842,)
.
augmented_samples_distribution = cnt_img_in_classes(y_train) diagram(augmented_samples_distribution)

, . keras.utils
to_categorical
, y_train
t_test
, one-hot encoding.
y_train = to_categorical(y_train, 43)
y_test = to_categorical(y_test, 43)
Keras]. , CNN ( ). CNN .
:
2 Conv2D (filter=32, kernel_size=(5,5), activation=”relu”)
MaxPool2D ( pool_size=(2,2))
Dropout (rate=0.25)
2 Conv2D (filter=64, kernel_size=(3,3), activation=”relu”)
MaxPool2D ( pool_size=(2,2))
Dropout (rate=0.25)
Flatten , 1
Dense (500, activation=”relu”)
Dropout (rate=0.5)
Dense (43, activation=”softmax”)
class Net:
@staticmethod
def build(width, height, depth, classes):
model = Sequential()
inputShape = (height, width, depth)
if K.image_data_format() == 'channels_first':
inputShape = (depth, heigth, width)
model = Sequential()
model.add(Conv2D(filters=32, kernel_size=(5,5), activation='relu', input_shape=inputShape))
model.add(Conv2D(filters=32, kernel_size=(5,5), activation='relu'))
model.add(MaxPool2D(pool_size=(2, 2)))
model.add(Dropout(rate=0.25))
model.add(Conv2D(filters=64, kernel_size=(3, 3), activation='relu'))
model.add(Conv2D(filters=64, kernel_size=(3, 3), activation='relu'))
model.add(MaxPool2D(pool_size=(2, 2)))
model.add(Dropout(rate=0.25))
model.add(Flatten())
model.add(Dense(500, activation='relu'))
model.add(Dropout(rate=0.5))
model.add(Dense(classes, activation='softmax'))
return model
Adam
, — categorical_crossentropy
, . model.fit()
.
epochs = 25
model = Net.build(width=30, height=30, depth=3, classes=43)
model.compile(loss='categorical_crossentropy', optimizer='adam', metrics=['accuracy'])
history = model.fit(X_train, y_train, batch_size=64, validation_data=(X_test, y_test), epochs=epochs)

, 25 93% . matplotlib
.
plt.style.use("plot")
plt.figure()
N = epochs
plt.plot(np.arange(0, N), history.history["loss"], label="train_loss")
plt.plot(np.arange(0, N), history.history["val_loss"], label="val_loss")
plt.plot(np.arange(0, N), history.history["accuracy"], label="train_acc")
plt.plot(np.arange(0, N), history.history["val_accuracy"], label="val_acc")
plt.title("Training Loss and Accuracy")
plt.xlabel("Epoch")
plt.ylabel("Loss/Accuracy")
plt.legend(loc="lower left")
plt.show()
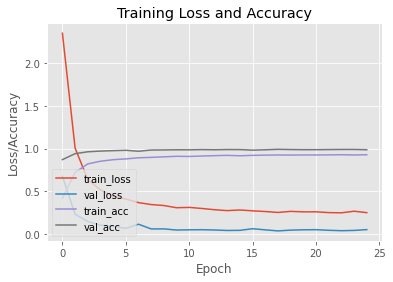
データセットには「Test」フォルダTest.csv
が含まれ、ファイルには画像パスとクラスラベルに関連する情報が含まれています。Test.csv
フレームワークを使用して、ファイルから画像とラベルへのパスを抽出しますPandas
。次に、画像のサイズを30x30ピクセルに変更し、numpy
すべての画像データを含む配列を作成します。accuracy_score
fromを使用して、sklearn metrics
モデルの予測の精度を確認します。このモデルで96%の精度を達成しました。
y_test = pd.read_csv('Test.csv')
labels = y_test["ClassId"].values
imgs = y_test["Path"].values
images=[]
for img in imgs:
image = Image.open(img)
image = image.resize((30,30))
images.append(img_to_array(image))
X_test=np.array(images)
pred = model.predict_classes(X_test)
print(accuracy_score(labels, pred))
0.958590657165479